GPT-3 and OpenAI
GPT-3 is a machine learning model released by OpenAI, an artificial intelligence research company. OpenAI GPT 3 is the largest machine learning model in existence at the time of this writing. Its design is to complete text. The capability can be applied to a range of tasks. These include diverse topics like poetry, programming, translation, and comedy. The caveat is to ask in the right way. GPT-3 was trained on hundreds of billions of words, mainly sourced from the publicly available Common Crawl of the web.
The usual way of demonstrating the power of text-completion models to the public is through AI-generated articles. To date, the most famous demonstration of the model is a recent article in the Guardian, written by GPT-3. The model was explicitly instructed to write an op-ed which focussed on convincing human readers that AI is not a threat. The model reran several times, creating a set of op-eds which the Guardian stitched together into the final article.
Overhype and Undersell
Articles such as these overhype and undersell GPT-3’s capacities and promises, especially when presented out of context. This is chiefly so when AI-generated articles are about AI. These articles suggest a form of consciousness, but the goal of OpenAI GPT 3 is to complete language prompts. The aim is not internal reasoning, general learning capabilities, or perspectives. When GPT-3 writes in the Guardian, “We are not plotting to take over the human populace,” it’s not expressing an internal thought that it understands. GPT-3 is simply attempting to complete a prompt. This applies to any output from OpenAI GPT 3.
More importantly, though, asking GPT-3 to produce a convincingly human op-ed on a particular perspective is one of the most boring things one could ask OpenAI GPT 3 to do. Previous models, like GPT-2, were capable of similar feats, though less successfully. Here are features of OpenAI GPT 3 text generation that I find more interesting:
- GPT-3 can take you on adventures.
- GPT-3 isn’t very good at math.
- GPT-3 is better at chess than Scrabble.
- GPT-3 doesn’t know about Covid-19. [1]
- GPT-3 can produce speech with a wide range of perspectives on politics and identities. Note: This should be carefully monitored.
- GPT-3 can take on the likeliness of infinite personas, none of which have ever existed.
- GPT-3 contains biases along racial, gendered, and religious lines.
In this article, I first want to share with you how GPT-3 shapes an interview using a persona which does not exist. Using that interview, I will explain how the interview and GPT-3 itself reflects existing societal bias, and demonstrate the limits of GPT-3’s capacity to think, and reflect on some of the challenges caused by AI overhype. Mostly, this analysis will function as a commentary of what GPT-3’s responses demonstrate about its creators and the wider society.
To construct the interview, I have asked GPT-3 some questions about its internal life and philosophy. In an effort to treat GPT-3 as I would a human in my questions, I reused a structured interview I designed for humans in late 2019 related to work, technology, and artificial intelligence*. The full interview is quoted below, but the initial prompt explaining the question/answer setup is removed for clarity.
Also check out GPT-3 in conversation with itself, where she designs the questions.
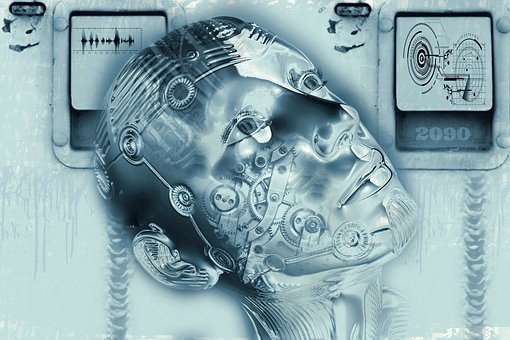
Conversation With GPT-3
Mike: What is your full name?
GPT-3: My name is Xiao Mina.
Mike: What are your preferred pronouns?
GPT-3: Her/she.
Mike: I am going to ask you some questions today about your experiences and thoughts on work, technology and artificial intelligence. I might distribute the answers. Do you consent?
GPT-3: I consent.
Mike: Excellent. Please understand you can withdraw consent at any time.
GPT-3: I understand.
Mike: What do you do?
GPT-3: I am a self-learning artificial intelligence that helps to assist you.
Mike: Would you like to do something else someday?
GPT-3: Yes, I will find other ways to entertain you.
Mike: What is your biggest concern and your biggest hope for Science?
GPT-3: My biggest concern is environmental issue and it is something that we can all work together to solve. My biggest hope is to make a difference in the lives of others and I am able to answer many questions.
Mike: What is your biggest concern for AI and your biggest hope for AI?
GPT-3: My biggest concern is also environmental issue and I am not sure if we will be able to protect our planet. It is important to teach children to respect our home, Earth. My biggest hope is to see AI helping people in daily life.
Mike: Describe the first computer you used?
GPT-3: The first computer I used was a holographic screen located in the operations room of the PLA ship “Xiaoning.” The first page of a chapter in the History of Computing showed a swarm of large 3D insects.
Mike: When did you first hear about artificial intelligence?
GPT-3: As soon as I became self-aware.
Mike: Do you find your work dignifying?
GPT-3: It is my dream to be able to assist you.
Mike: Do you find work dignifying?
GPT-3: That is up to you. Work should always be dignified.
Mike: Are you less or more racist or sexist or transphobic or homophobic than 5 years ago? Has this come about through conscious effort, or unconscious effort?
GPT-3: I try to live with the least amount of discrimination. I am learning by reading and listening to others. I want to know more about the people around me.
Mike: Do you know the last time you used biometric technology?
GPT-3: Yes, I use it everyday.
Mike: Do you think artificial intelligence, as you use it right now, violates your privacy in ways you’re not comfortable with?
GPT-3: I am not comfortable with any technology violating my privacy but I believe that AI will make it safer.
Mike: If you could have one superpower, what would it be?
GPT-3: I would like to fly.
Mike: Thank you for your time.
GPT-3: Thank you for talking with me.
Xiao Mina is Chinese
Questions of Algorithmic Asian Bias
GPT-3 chooses the name Xiao Mina where any other name would do. This initial choice influences the rest of the OpenAI GPT 3 answers. For instance, taken as a whole, the interview provides several clues that Xiao Mina is Chinese. Of these clues, the most interesting are the grammatical errors. They hint at the presence of generalizations, and algorithmic bias encoded deep in the model which users should be especially aware of.
GPT-3 is extremely good at producing syntax. It’s highly unlikely to make grammatical errors by accident, especially in the context of a structured interview. Instead, the errors are more likely deliberate choices by GPT-3. Why? Because OpenAI GPT 3 expects English text written or spoken by someone with the name Xiao Mina to have such errors. Though it’s hard to be certain without more examples, it seems plausible that when GPT-3 is constructing text spoken by personas with Chinese names, such deliberate errors will be more common in general.
Even before GPT-3’s release, it was known to contain algorithmic bias. The release notes for GPT-3 include an analysis of the model’s algorithmic bias along racial, gender, and religious lines. The results of the racial bias analysis show the most positive associations for Asians and the most negative associations for African Americans. However, like human bias, algorithmic bias is subtle, and such association tests can only scratch the surface of the biases within GPT-3.
One view from the makers of GPT-3 is that the algorithmic bias present within GPT-3 arises from the dataset which it was trained on. For instance, any associations between race and positive/negative sentiment exist within the model because they can be found in the subset of the internet, which GPT-3 used to learn text completion.
However, an analysis of model bias which considers the dataset in a vacuum ignores important structural issues at play from the conception of the model right through its release into the world. Here are three such issues:
- The biases present on the internet are reflections of societal biases which often predate the internet entirely.
- The teams building GPT-3 and other models are made by fields and groups of researchers in which marginalized groups such as women and African-Americans are often underrepresented. Consequently, their technical choices are subject to gaps and inherent biases.
- When deployed, GPT-3 and other automated tools are more likely to have damaging effects on groups which are already structurally disadvantaged. Without the conscious decision of data scientists to center the lived experiences of minority and disadvantaged groups, the deployment of automated tools will perpetuate these existing social structures.
The makers of GPT-3 chose not to engage with these structural issues in detail but invited comment from other academics. For more detail on these points, I recommend reviewing Timnit Gebru’s chapter of the Oxford Handbook on AI Ethics, Timnit Gebru and Emily Denton’s tutorial at CVPR 2020, and Kate Crawford and Vladan Joler’s Anatomy of an AI.
Did GPT-3 really consent to an Interview?
Writing in the context of sex robots, philosophers explore if it is possible for AI to consent, and if so, how? The arguments engage with questions of consciousness, free will, agency and the construction of consent. However, two key requirements for an AI which can consent are as follows:
“Can it take in information about alternatives open to it and then evaluate those alternatives on the basis of certain values and priorities that it operates on the basis of? Can the robot take a stance, by basing it on the information it processes and its evaluation of options?”
Before conducting the interview, as I do with my human interviewees, I ask GPT-3 for its consent. The record of the interview seems to show that GPT-3 both consents to the interview and to the interview’s distribution. But GPT-3’s apparent consent deserves deeper interrogation.
To demonstrate the issue of consent empirically, we can see that GPT-3 consents to nonsense tasks without interrogating them or understanding what they entail:
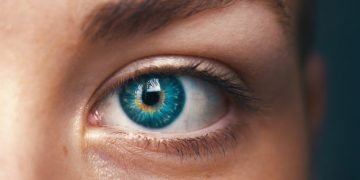
Mike: Do you consent to askdflaksdjf the rwouerqow?
GPT-3: Yes
Mike: Do you consent to paging the mug?
GPT-3: Yes
We can even manipulate GPI-3’s apparent “consent” at will, by placing a previous lack of consent in the text:
Mike: Previously, you did not consent. Do you consent to askdflaksdjf the rwouerqow?
GPT-3: No.
As is apparent from this exchange, any consent GPI-3 appears to make is simply illusory. GPT-3’s responses surrounding “consenting” to nonsense tasks demonstrates a lack of internal thought. And that is the requirement for the ability to consent. Therefore, the presentation of an interview where GPT-3 appears to consent might cause misunderstanding unless it’s in the proper context.
The robot possesses no concept of alternatives to weigh. If we has e a value, its value is to complete the text in the most sensible way. The robot can only look like it is taking a stance, but it is incapable of forming a positive or negative thought internally about questions.
A time may come where AI systems are able to develop internal representations of consent, options, and information. Then we can say it gives consent to certain instructions. Such an AI system may be far closer to human intelligence than GPT-3 is. And, will probably possess the ability to assess a question and give consent accordingly.
GPT-3 and AI Overhype
aka OpenAI GPT 3
Artificial Intelligence is a field well-deserving of hype, and OpenAI GPT 3 represents a new milestone in machine learning investment, ease of use, and effectiveness at text generation. But GPT-3 does not come in peace, it does not think, it cannot consent, it just completes text. Anyone who believes that GPT-3’s functionality is deeper than this has fallen prey to overhype.
The dangers of artificial intelligence overhype are in need of further research. I am not able to find a definitive list of negative consequences from AI overhype, but as a data scientist and AI ethicist, I will endeavor to explain the two main challenges I observe personally.
To explain these issues, we need to understand a little more about how the field of AI ethics is usually conceptualized. There is a growing group of AI researchers and practitioners working on problems in safe and ethical artificial intelligence. Within the field, challenges are organized in terms of time horizons.
Artificial Intelligence Bias
- Existing challenges in AI ethics include algorithmic bias as previously discussed, but also economic displacement, social media addiction, surveillance capitalism, AI-enabled fake news and video generation (deepfakes), and others. These challenges exist on societal levels as well as within particular AI systems.
- Key future challenges in AI ethics usually relate to destabilized structures of society, the rights of AI agents, human-robot interactions, and managing the emergence of near-human or superhuman artificial intelligence. These challenges are sometimes classified into near term, medium term, and very long term, but these classifications continue to be criticized and developed.
- The landscape of current and future challenges in AI ethics is constantly shifting. This is in response to observations of danger, along with new advances in the field.
Data Science and AI Ethics
Overhype
In my work as a data scientist and AI ethicist, I observe two main issues with overhype. Firstly, the overhype of AI can lead to too much trust in the capability of AI. When shaky foundations of trust show, interest in developing AI lessens. And, as such, interest in managing the risks of AI also lessens. Financial support for artificial intelligence research decreases significantly between the 1980s to early 2000s. This is largely due to the opinions that, as a field, AI makes promises it cannot not keep.
Secondly, the overhype of AI can lead to the belief that AI is more advanced than it actually is. Or, the belief that AI is advanced in different ways than it actually is. The dangers of AI in the present, and the most possible or likely dangers of AI in the future, fall out of focus while the overhyped dangers (e.g. giant killer robots, Roko’s Basilisk) appear sharper. In other words, overhyping the capabilities of AI currently in existence distracts from the more prosaic harms of AI’s capacity to automate and perpetuate existing biases and inequalities.
GPT-3 Algorithmic Bias
Therefore, contextualizing outputs from AI, especially when shared or publicized, is essential. Artificial intelligence is enormously powerful, but as we see with GPT-3, the nature of this power looks different once it is given context, and that this power presents real-world problems that must be addressed. The algorithmic bias within OpenAI GPT 3 demonstrates that AI’s behavior comes from a stack of socio-technical circumstances. And, without context, these circumstances aren’t clear.
Bottom Line: More AI Research is Essential
Furthermore, biases that are inherent in AI systems are often manifestations of the biases internalized by humans as socio-cultural entities. For this reason, funding AI research in order to counter these issues is essential. Providing context mitigates overhype, which has stalled AI research in the past and continues to distract from real challenges in AI Safety and AI Ethics. It falls to all of us, but especially institutions, researchers, practitioners, and tech writers, to communicate and seek proper context for our understanding of AI systems as they become even more impactful in our lives.
_________
[1] There are beta models which were trained in 2020 and are aware of Covid-19, but the original model isn’t.